Articles
A Healthcare System Perspective on AI
We shouldn't confuse "Artificial Intelligence" with "useful."
12/08/2022
by David Gaines
It looks like Artificial Intelligence (AI) will continue to be the hot topic in the business and technology world for quite a while, so I'll wade in with a framework and some hot takes... Most of my expertise is in healthcare data, and my thoughts here are health plan / healthcare administration-specific. However, much of what I say is going to apply to other fields.
Framework: While AI is all the rage, the presence of AI doesn’t, on its own, ensure a product will deliver value. AI’s usefulness is domain-specific and depends on the input data, how well that input data aligns with with the questions we want answered, and how little error is tolerated in that answer. A simple framework for evaluating an AI product is to ask, what specific “capabilities” does AI enable, and what’s the “efficiency” with which it does the work that a human would otherwise do?
If the data isn’t structured correctly or specifically enough in a domain, AI is at risk of giving you obvious or useless and unexplainable outputs.
One of the reasons ChatGPT is so impressive is because 1) it leverages a data set of all publicly available writing over all of human history and 2) researchers around the world have spent 70+ years figuring out how to digest, structure, restructure, and abstract text information in a manner that computers can use to create useful outputs, including those resembling how a decently-knowledgeable person would answer a question or produce a writing sample. Even then, it took thousands of people years to train the ChatGPT model.
Most industries, however, have neither the data nor data model advantages, and have extremely high standards for accuracy and precision where “decently-knowledgeable” wouldn’t cut it.
I run a data engineering and analytics company in healthcare. We take variable and fractured data from health plans and do a lot of novel work to make the data structured in a way that creates a relevant, use-able, and useful record of what happened in that health plan.
I've been 100% guilty of using the "AI" label as a lazy and over-hyped signal rather than describe the complex web of judgement, knowledge, and statistics my company relies on for our work. Some of the methods fall under the umbrella of “AI”, others do not. I use the “AI” label more frequently because it is useful shorthand and common in marketing.
Most of the useful stuff our company does today results from getting our computer to perform logic based on leveraging painstaking research and human reason. Looking at statistical relationships (the stuff most people call “AI”) is part of it, but it’s more for quality assurance than anything else. Most of the useful analytics our company does today is comparing averages, like the difference in unit costs between two facilities. Useful averages don’t exist in our industry because the underlying data is broken; we don’t have industry-wide standard definitions of what a healthcare service or “unit” (i.e. an MRI) is from historical data. See this article if you want more details.
In my industry, anyone who tells you healthcare administration (i.e. cost, resource allocation, networks, efficiency, etc.) is going to be solved by AI is glossing over some important details. Other than our company (shameless plug), almost no one can comprehensively tell you how much most individual healthcare services or decisions cost from historical data. That means there’s no data to be leveraged to train a computer to see or recommend useful and actionable insights for improving decisions that manage costs. Look no further than our current healthcare system for proof…
Here's a picture from our platform of the spread of diagnostic colonoscopy and chest x-ray prices for a commercial health plan in one month in 2022. Each bar represents the price of a Careignition-normalized unit of purchase.
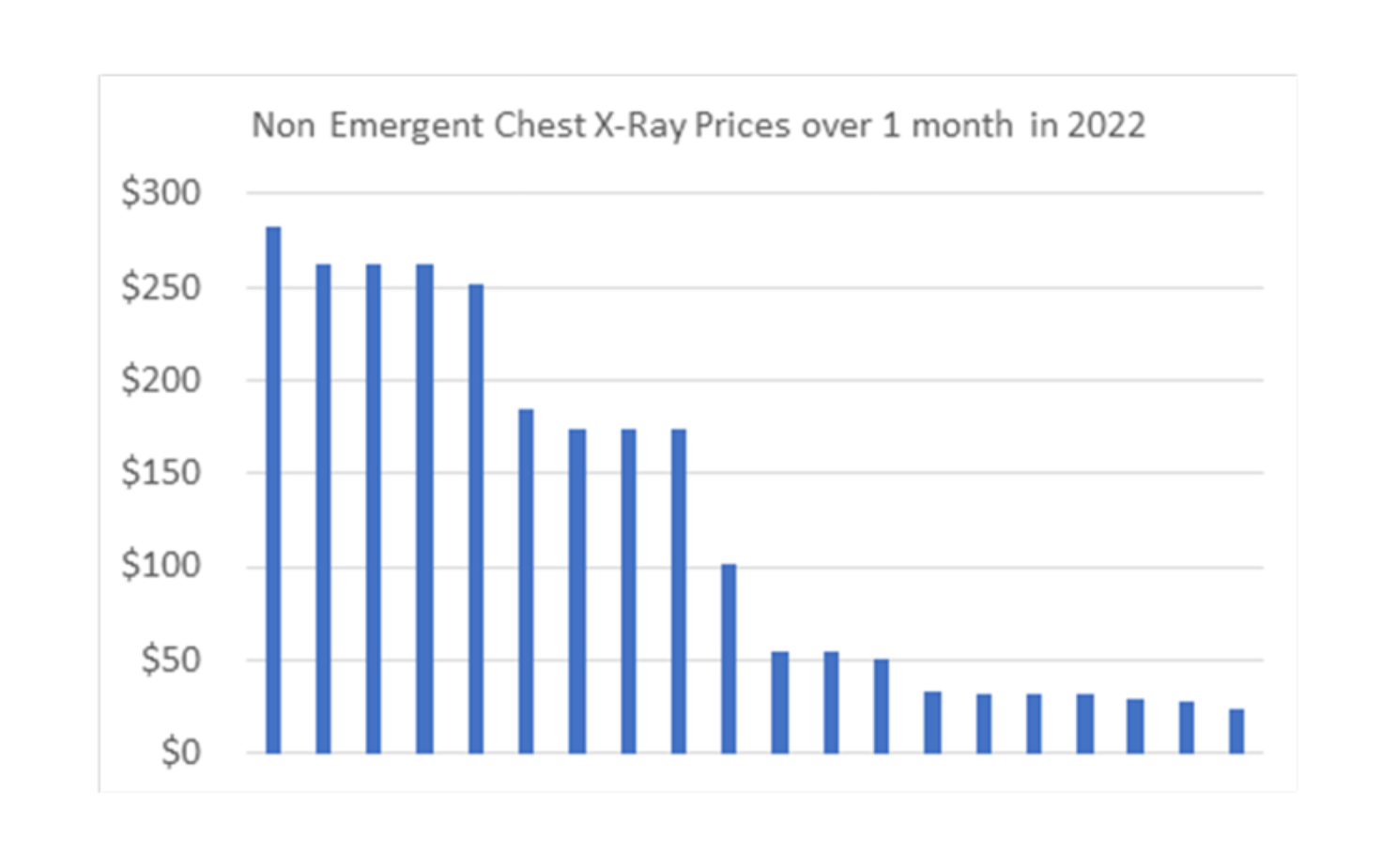
.png)
The issue expressed by these graphs is the most glaring, first-order problem of our healthcare system today. Healthcare costs too much, we don’t allocate it well, and there is no accountability when one party is paid 15-times another for the same thing. We haven’t solved this problem and “AI-powered” analytics companies don’t report accurately report on it… People are too busy focusing on the future of AI to figure out the issues today.
Which is what leads me to reiterate, most AI for decision support in the healthcare economy (allocating patient volume and attention) is not very useful today.
Here is a real example from analytics leaders in our space: In a presentation, they showed how they use AI to predict future outcomes so you can intervene to lower costs. The example they presented was how the “AI” predicted that a 98 year-old who has recently gone to the hospital has a high probability of mortality within the next year. The AI’s recommendation is that someone should go to that person and try to get them to go to hospice to avoid readmission to the hospital.
- Do we really need AI to tell us that a 98-year-old who just went to the hospital doesn’t usually have much time left to live?
- Are we sure that telling a 98-year-old we have given up on them and put them in hospice is good, moral, or effective idea? I bet that 98-year-old, even if his mortality was accurately predicted (it may not be), would want to spend his last days his way. Readmission rates be damned.
There are a lot of things like this in healthcare that may be predictable but are otherwise 1) obvious or 2) not change-able, or 3) not cost effective when impacted. People like predicting hospital re-admissions and ER admissions, but there is limited evidence that these metrics can be cost-effectively impacted. It’s also not clear to me that these are the most important metrics when it comes to making sure people receive valuable healthcare.
Now, why was this case (and those like it) used as an example of “cutting-edge” AI? Because the input data is set up to predict these outcomes, and it is a good excuse to use fancy AI computer programs. There are easy to find variables of “age”, “death”, “diagnosis”, and “hospital admission” (see article here). So getting an output of X% chance of death, given a set of conditions is pretty straightforward and compute-able. Just because we can apply “AI” prediction models to the problem doesn’t mean it’s useful.
Now, answering a more useful question like: “given a patient, what is the optimal location to allocate them for a diagnostic chest x-ray before a surgical procedure?” is a lot harder, because historical healthcare data today does not have a structure that reflects:
1) A clear price for that chest x-ray,
2) A clear picture of where chest X-rays have happened in the past (because there can be multiple parties at different locations involved),
3) An understanding of that a chest x-ray is not the only pre-procedural service someone will undergo before a surgery,
4) The patient’s affiliated costs (i.e. drive time),
5) The downstream causal relationships between one provider and another, and
6) Doesn’t know if the eventual surgeon will even accept the pre-procedural information…
If the information necessary for a person to solve the problem isn’t present in the data. A computer probably can’t figure it our either.
For AI magic to take place in healthcare or other domains, there needs to be a large investment in the information structure, so that a computer can help us make decisions we care about. You need specific knowledge and care, not correlations, to structure data properly for this purpose; once you do that, then AI can work its magic, but that first step is hard and not nearly as scalable for healthcare as AI evangelists may proclaim.
This pattern exists in many industries, and I think for entrepreneurs, investors, and purchasers being sold the wonders of “AI” it makes sense to think about what useful problems there are to solve, and whether the existing data is actually set up for a computer to help find a solution. So, when someone tell you that you need to buy or invest in the latest and greatest AI-powered product, it's really important to pull back the curtain and try to understand whether they actually do that and, if so, how. If AI’s outputs are not reasonable recommendations that line up with what people can actually do, or if they are not based on well-structured information that a smart analyst can reason with, don’t believe the hype.
Let's get started
Learn how Careignition can illuminate your health data.